Member $50.00 | Non-Member $150.00
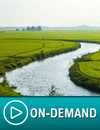
View Important Policies and System Requirements for this course
INSTRUCTORS:
Bryan Osborne
Kushal Adhikari, Ph.D.
Michael Gregory, P.Eng, P.E.
Kayla Brown
Dong Hee Kang
Zhengxiao Yan
Ryan Johnson, Ph.D.
Sierra Young
Andrew Erikson, P.E.
Anjulie Cheema, P.E.
Course Length: 2 hours
Purpose and Background
These presentations were recorded at the 2024 World Environmental & Water Resources Congress:
Water Quality Assessment of Muddy Run, Pennsylvania, USA (11 minutes)
This presentation detailed a comprehensive study conducted at Juniata College, where various water quality parameters such as temperature, pH, dissolved oxygen, and nutrient levels were measured across multiple sites and dates. It highlighted the impact of urbanization and culverting on water quality, demonstrating significant variations in these parameters due to human activities. The findings emphasized the need for further research to understand the long-term effects of these factors and suggested potential remediation strategies. This study provides valuable insights into the challenges and complexities of maintaining water quality in urbanized watersheds.
Clarifying the Clarity of Dirty Stormwater (17 minutes)
This presentation delves into the intricacies of modeling and managing suspended solids in stormwater runoff. It discusses the importance of breaking down pollutants into various mass fractions and analyzing their distinct settling velocities to accurately model their behavior in water systems. The technical content emphasizes the need for precise measurement and classification of particle sizes to understand their impact on water quality and sediment transport. By leveraging tools like EPA SWIM 5, the presentation highlights advanced methods to simulate and improve stormwater management practices.
Implementing AI on Image Processing for Stormwater Control Measure Using Unmanned Aerial Vehicle-Acquired Imagery (13 minutes)
This presentation explores the use of AI and drones to enhance stormwater management. It details the application of AI techniques, including YOLOv8 and RoboFlow, for object detection, image segmentation, and classification to identify stormwater control measures and trash in UAV-acquired images. The content highlights the development of effective methods for evaluating stormwater systems, leveraging machine learning to analyze drone-captured images and improve water quality. The research underscores the potential of AI to optimize stormwater management and streamline debris removal processes.
Evaluating the Effects of Climate Change on Harmful Algal Blooms in Coastal Estuaries Integrating Data-Driven and Downscaling Approaches (16 minutes)
This presentation explores the application of game theory and stochastic methods to optimize pollution control strategies in river systems. It details the development of a model that simulates the interactions between various stakeholders, incorporating uncertainties in pollutant sources and environmental responses. The approach provides a framework for evaluating cooperative and competitive scenarios, aiming to achieve cost-effective pollution reduction. Additionally, the presentation examines how this model can inform the design of regulatory policies and incentive mechanisms to enhance water quality management.
Evaluating the Effects of Climate Change on Harmful Algal Blooms in Coastal Estuaries Integrating Data-Driven and Downscaling Approaches (16 minutes)
This presentation explores the use of AI and drones to enhance stormwater management. It details the application of AI techniques, including YOLOv8 and RoboFlow, for object detection, image segmentation, and classification to identify stormwater control measures and trash in UAV-acquired images. The content highlights the development of effective methods for evaluating stormwater systems, leveraging machine learning to analyze drone-captured images and improve water quality. The research underscores the potential of AI to optimize stormwater management and streamline debris removal processes.
Coupling Machine Learning with the National Water Model to Account for the Impacts of Water Resources Infrastructure: Improving Streamflow Prediction Skill for Great Salt Lake Management (13 minutes)
This presentation focuses on enhancing the accuracy of streamflow predictions. It addresses the limitations of the National Water Model, particularly its inability to account for water resource infrastructure like reservoirs. The study integrates machine learning techniques, including XGBoost and deep learning models, to correct the discrepancies between the model's predictions and observed data. This approach aims to improve water management strategies by providing more reliable forecasts for the Great Salt Lake area.
Advancing Camera-Based Monitoring for Operational Water Resource Applications (15 minutes)
This presentation explores the use of camera systems to enhance hydrologic monitoring. It focuses on leveraging existing camera networks and newly installed systems to collect visual data for assessing streamflow and water levels. The project integrates deep learning and computer vision techniques, such as image segmentation and velocity estimation, to extract meaningful hydrologic information from camera footage. Key challenges addressed include accurate water segmentation in natural channels and handling nighttime imagery for continuous monitoring.
Maximize the Effectiveness of Existing Wet-Weather Infrastructure through Operational Optimization (14 minutes)
This presentation discusses the use of advanced optimization techniques to enhance the performance of existing wet-weather infrastructure. The approach involves leveraging the "Optimizer" software platform to explore a wide range of real-time control strategies for combined sewer overflow (CSO) management. The study emphasizes balancing the reduction of CSO volume with the potential risk of system surcharge or flooding, using detailed simulations and data analysis to find optimal solutions. The outcome demonstrates significant CSO reduction without new construction, highlighting the efficiency of real-time adjustments and system optimization.
Benefits and Learning Outcomes
Upon completion of this course, you will be able to:
- Identify key challenges and potential strategies for maintaining water quality in urbanized watersheds based on the findings from the Muddy Run study.
- Describe the process of classifying and modeling suspended solids in stormwater using different mass fractions and settling velocities.
- Explain how AI techniques like YOLOv8 and RoboFlow can be used for object detection and classification in UAV-acquired imagery for stormwater management.
- Identify the key climatic and environmental factors that influence harmful algal blooms in coastal estuaries, as analyzed through data-driven approaches.
- Explain how machine learning techniques like XGBoost and deep learning models enhance streamflow predictions by accounting for water resource infrastructure.
- Describe the use of deep learning and computer vision techniques, such as image segmentation and velocity estimation, in extracting hydrologic information from camera footage.
- List the potential benefits of using real-time control strategies and optimization software, like the "Optimizer" platform, in reducing CSO volumes and managing system risks.
Assessment of Learning Outcomes
Learning outcomes are assessed and achieved through passing a 10 multiple choice question post-test with at least a 70%.
Who Should Attend?
- Water resource engineers
- Consulting engineers
- Utility engineers
- Public Agency Engineers
- Utility Directors
How to Earn your CEUs/PDHs and Receive Your Certificate of Completion
This course is worth 0.2 CEU/2 PDHs. To receive your certificate of completion, you will need to complete a short on-line post-test and receive a passing score of 70% or higher within 365 days of the course purchase.
How do I convert CEUs to PDHs?
1.0 CEU = 10 PDHs [Example: 0.1 CEU = 1 PDH]