Member $249.00 | Non-Member $349.00
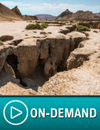
View Important Policies and System Requirements for this course.
INSTRUCTOR:
Zenon Medina-Cetina, Ph.D., M.ASCE
Co-Sponsored by ASCE Continuing Education and ASCE's Geo-Institute (G-I)
"The teleconference method allows the audience to see this webinar straight from my office, without having to travel. I have attended many ASCE webinars and find that ASCE knows how to put together an effective webinar." - Anonymous Attendee
Purpose and Background
This webinar is an introductory course on the proper applications of the Bayesian paradigm in engineering for improved decision-making within a Risk framework. The main aim is to provide key theoretical and computational resources to find the optimal use of evidence, including physical observations, model predictions and expert’s judgment, when confronted with the solution of an engineering problem.
This webinar is designed to follow a scenario-based approach, where a ‘true’ physical process is simulated after collecting basic evidence about it. Different scenarios are proposed for investigating the influence of varying evidence on optimal decision-making as applied in engineering practice. For instance, one scenario will replicate the case where a lead engineer has the option to collect some data about the process of interest, and to build a numerical model to simulate it, for making predictions beyond the sampling observations, and for proposing low Risk engineering solutions about it. The exercise will also define an experimental design that will take the web seminar student through a number of decision-making options typically found in engineering practice, such as making choices about surveying (location, amount, intensity, and frequency of samples), model development (competing models), and the hiring of experts’ advice.
In summary, the aim is to replicate common decision-making scenarios found in engineering practice when using the Bayesian paradigm, but by fully understanding its advantages and limitations, particularly in light of the competing theoretical inferences that each can (and cannot) generate.
Primary Discussion Topics
- Motivation for using an Uncertainty Quantification UQ approach within a Risk framework for improving decision-making in Civil Engineering processes
- Introduction to the Bayesian paradigm
- Introduction to the implementation of the Bayesian paradigm using Monte Carlo methods
- Discussion on a set of work examples to illustrate the advantages and limitations of Bayesian inference
- Introduction to the use of causality and probability via Bayesian Networks though simple applications
Learning Outcomes
Upon completion of this course, you will be able to:
- Enhance critical thinking skills for the optimal solution of engineering problems
- Examine the theoretical and computational advantages and limitations of the Bayesian paradigm
- Determine the outcomes from exploring solutions to the exercise’s problem using a Risk framework as a guiding strategy
- Assess the impact of key decision-making variables found during the course of an engineering project
- Assess the Impact on the definition of location and number of samples
- Assess the Impact of model complexity
- Assess the Impact of a given level of expert’s judgment
Benefits
- Students will benefit from setting a strong basis on the understanding of theoretical and computational Bayesian inference
- Learn about how to set up a probabilistic solution of an inverse problem or probabilistic calibration
- Explore future assessments of model performance, model selection, classification, forecasting and causal analysis on any engineering application
- Contrast Bayesian inference with its competing traditional approaches (the frequentist and the likelihoodist)
- Develop a solid foundation for the proper use of basic inference methods
- Be able to identify its advantages and limitations with respect to traditional curve fitting and optimization-based back-calculation approaches
- Relate not only the physical impacts of an engineering problem, but also the consequences, and the fragility or vulnerability of the engineering system, which would open the course to the participation of a broader spectrum of stakeholders
Assessment of Learning Outcomes
Students' achievement of the learning outcomes will be assessed via a short post-assessment (true-false, multiple choice and fill in the blank questions).
Who Should Attend
This webinar is oriented towards all decision-makers in engineering or relating fields, particularly those who value rational, systematic, and reproducible analysis of evidence; and those who value the use of Risk as a guiding index for improved decision-making.
Outline
- Motivation for a future Standardization of an Evidence-Based Approach for Improved Decision-Making within a Risk Framework
- Elements of Statistics and Probability
- Exercise
- Problem Definition
- Experimental Design: Physical, Simulation, and Expert’s Beliefs
- Solution of the Inverse Problem using the following approaches: (has three subbullets)
- Frequentist
- Likelihoodist
- Bayesian
- Elements of Inverse Theory: Bayesian and Tarantola’s
- Computational Statistics: Markov Chain Monte Carlo and Metropolis-Hastings
- Related Topics of Interest
- Causal Probability: Bayesian Networks
- Forecasting: Kalman Filtering and Bayesian Forecasting
- Sensitivity Analysis
How to Earn your CEUs/PDHs
To receive your certificate of completion, you will need to complete a short on-line post-test and receive a passing score of 70% or higher within 1 year of purchasing the course.
How do I convert CEUs to PDHs?
1.0 CEU = 10 PDHs [Example: 0.1 CEU = 1 PDH]